BigML Releases
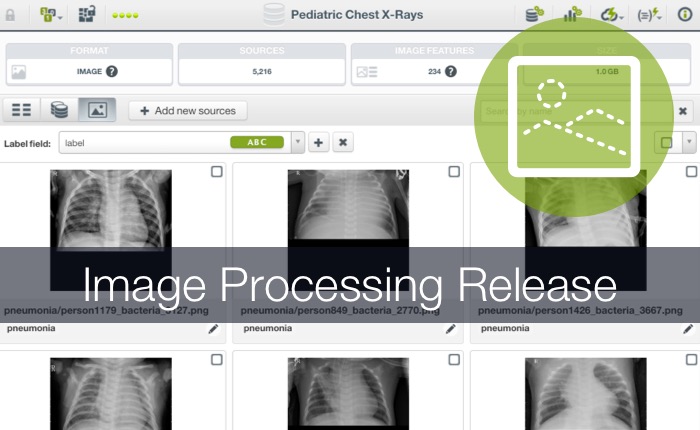
DEC 15 2021
Image Processing
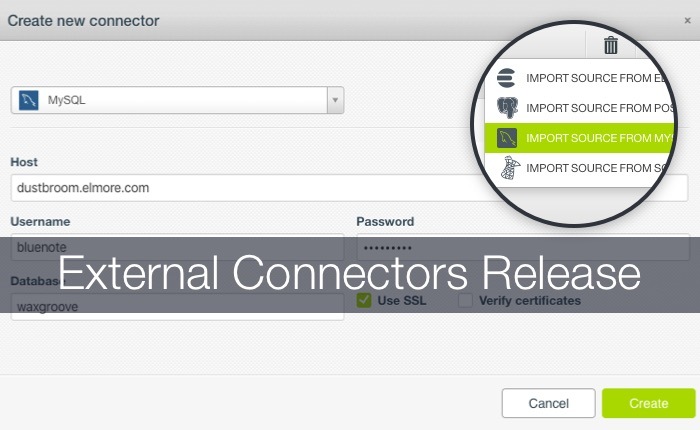
APR 16 2020
External Connectors
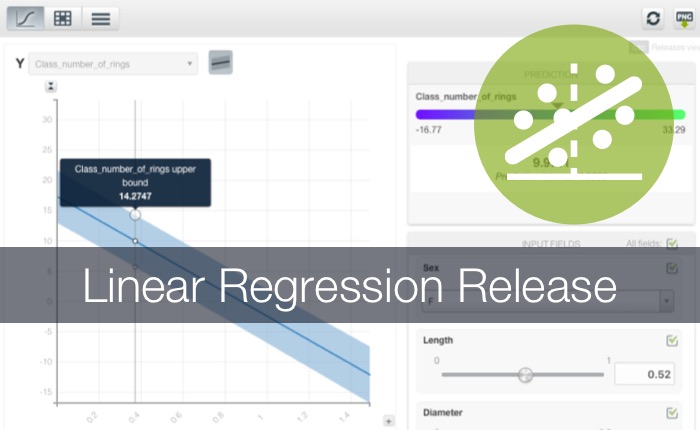
MAR 19 2019
Linear Regression
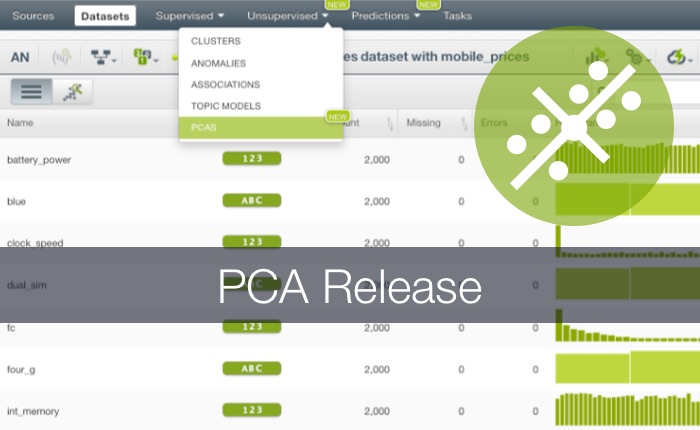
DEC 20 2018
PCA
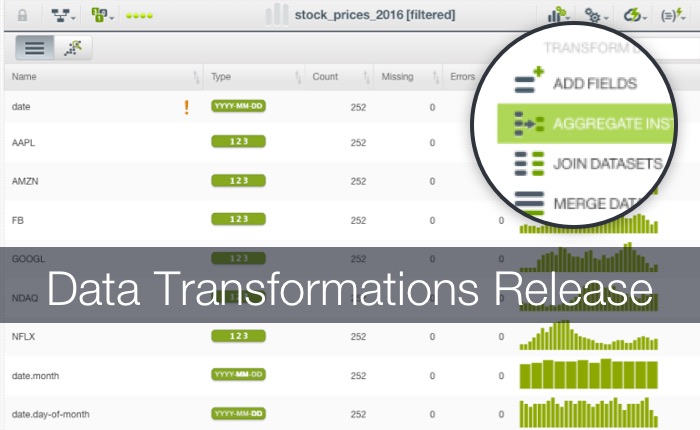
OCT 25 2018
Data Transformations
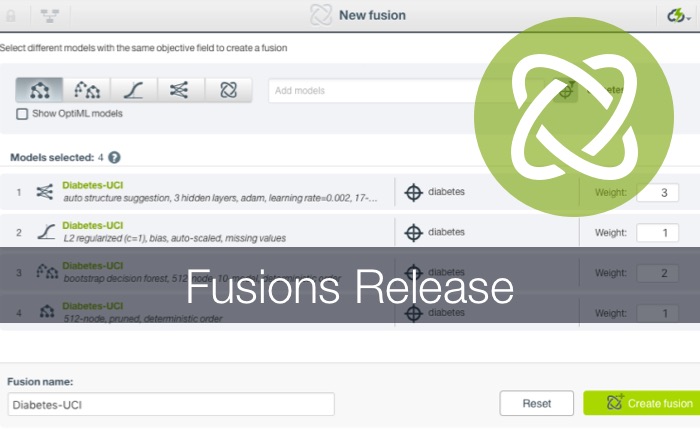
JUL 12 2018
Fusions
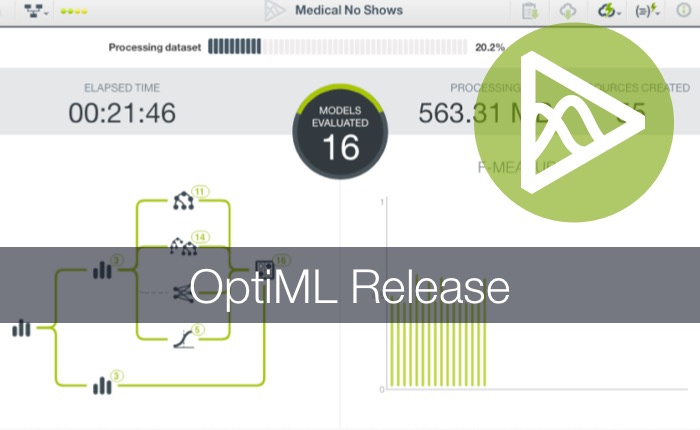
MAY 16 2018
OptiML
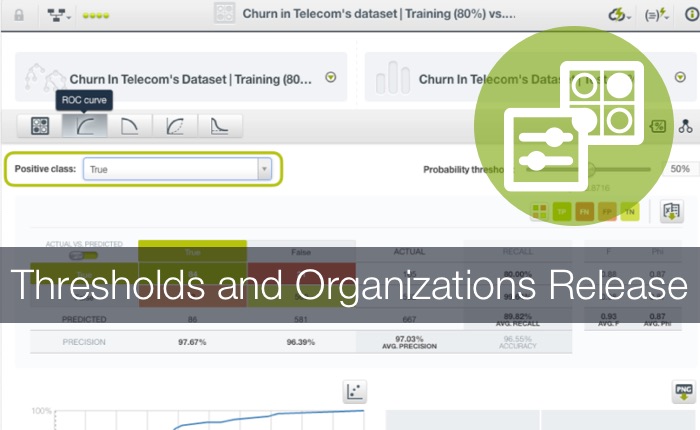
JAN 31 2018
Operating Thresholds and Organizations
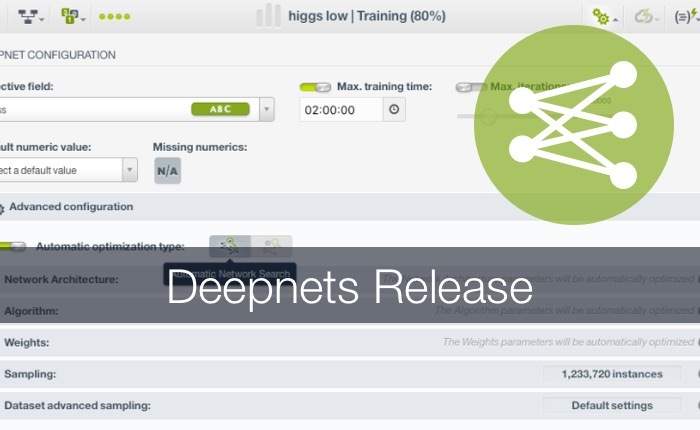
OCT 5 2017
Deepnets

JUL 20 2017
Time Series
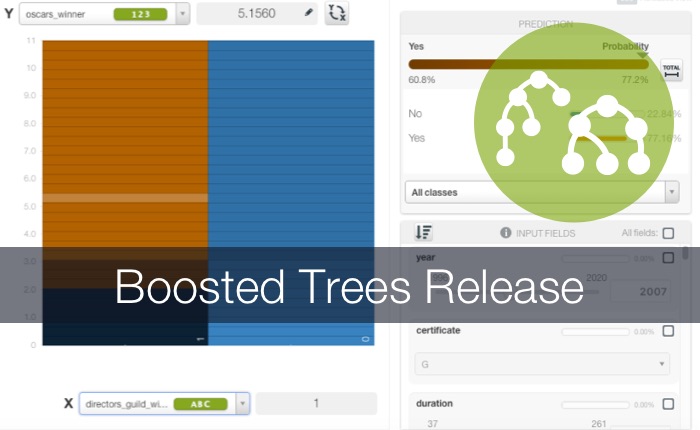
MAR 21 2017
Boosted Trees
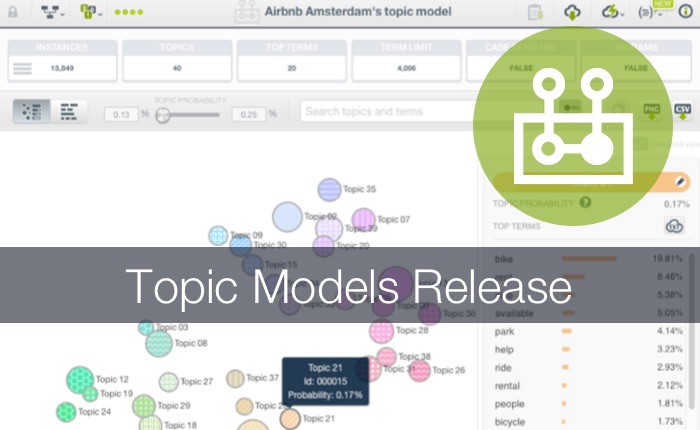
NOV 29 2016
Topic Models
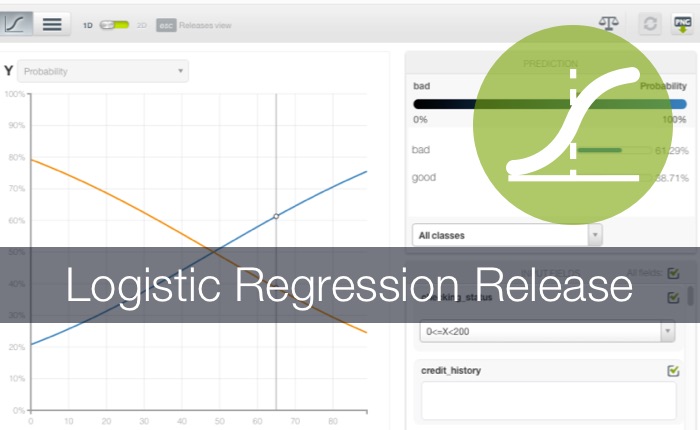
SEP 28 2016
Logistic Regression
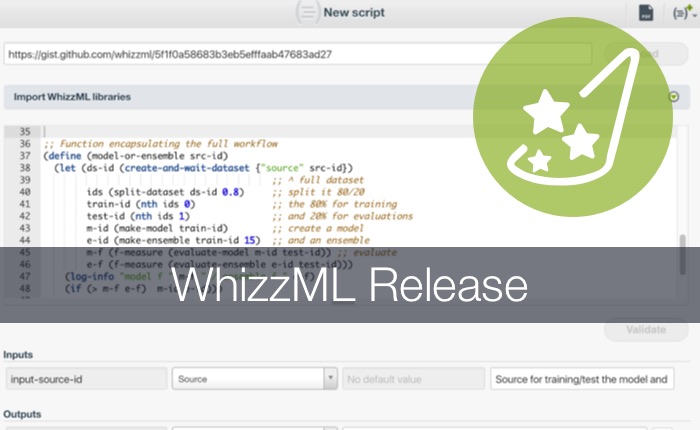
MAY 19 2016
WhizzML
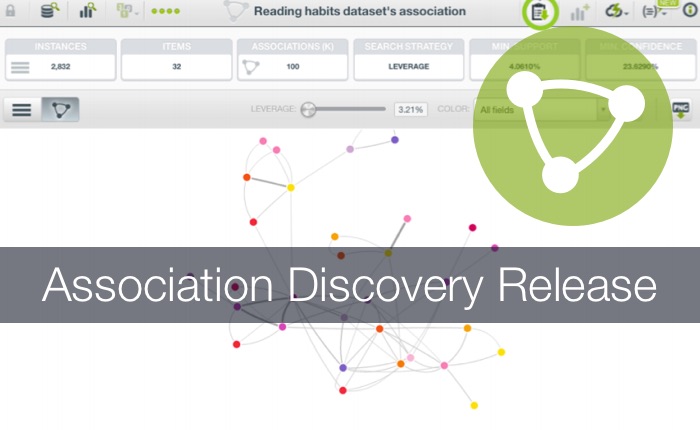
DEC 15 2015
Association Discovery
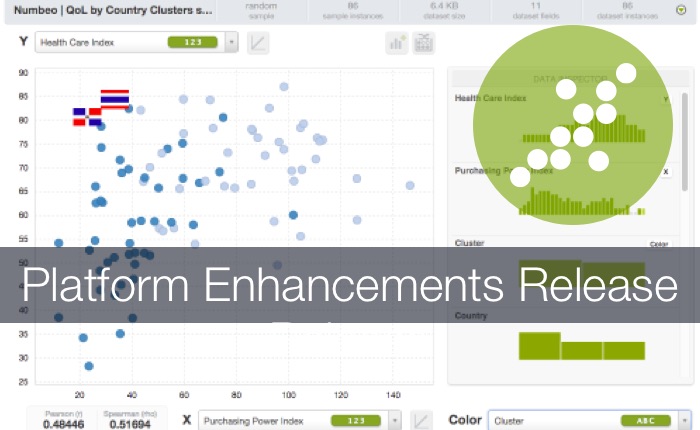
FEB 11 2015