Machine Learning made beautifully simple for everyone
BigML removes the complexities of Machine Learning so you can focus on what matters most, enhancing and automating decision making.
Comprehensive Machine Learning Platform
BigML provides a selection of robustly-engineered Machine Learning algorithms proven to solve real world problems by applying a single, standardized framework across your company. Avoid dependencies on many disparate libraries that increase complexity, maintenance costs, and technical debt in your projects. BigML facilitates unlimited predictive applications across industries including aerospace, automotive, energy, entertainment, financial services, food, healthcare, IoT, pharmaceutical, transportation, telecommunications, and more.
Supervised Learning: classification and regression (trees, ensembles, linear regressions, logistic regressions, deepnets), and time series forecasting.
Unsupervised Learning: cluster analysis, anomaly detection, topic modeling, association discovery, and Principal Component Analysis (PCA).
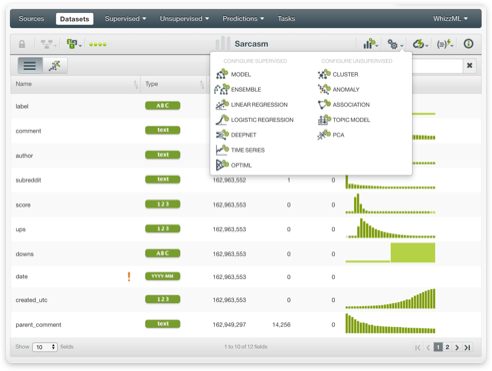
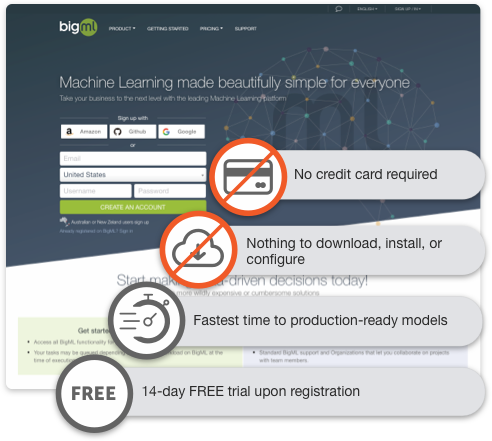
Immediate Access
Instant Machine Learning at your fingertips in the cloud or on-premises. Either way, you will be up and running with an easy-to-use web interface and REST API in a matter of seconds. Start your Machine Learning project today by simply signing up with your email — no sales demos, credit cards, or long-term contracts required.
Free Accounts: Latest full-featured version of BigML. Unlimited datasets and models. 14-day FREE trial upon registration with basic 2-tasks parallelization and 64Mb dataset size limit. No credit card required.
Prime Accounts: Boosted and professional higher tasks parallelization and dataset size limits. Flexibly adapted servers topology and prioritized job queues. Access to BigML Organizations, which let you collaborate on projects with team members.
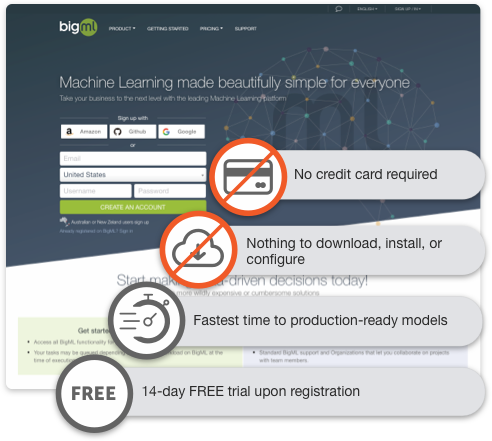
Interpretable & Exportable Models
All predictive models on BigML come with an interactive visualization and explainability features that make them interpretable. They can be exported and used to serve local, offline predictions on any edge computing device or be instantaneously deployed as part of distributed, real-time production applications.
Interpretable: Visualizations such as Partial Dependence Plots effectively generate and display thousands of model predictions at a glance while Prediction Explanations and Field Importances shed light on factors driving individual predictions.
Exportable: BigML models are fully exportable via JSON PML (and PMML) and can be used from all popular programming languages. This means you can seamlessly plug your models into your web, mobile or IoT applications or services such as Google Sheets, Amazon Echo, Zapier, and more.
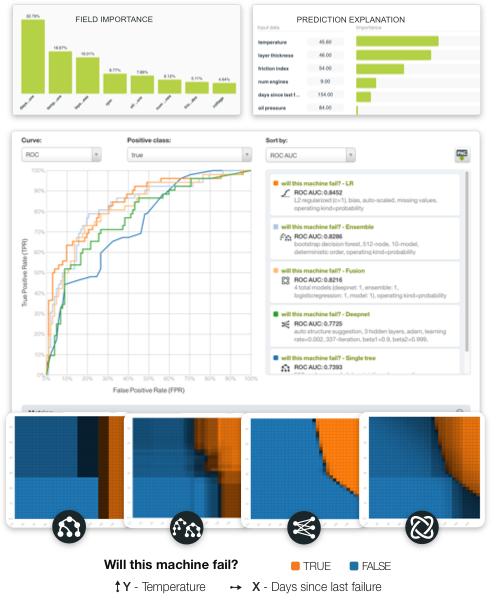
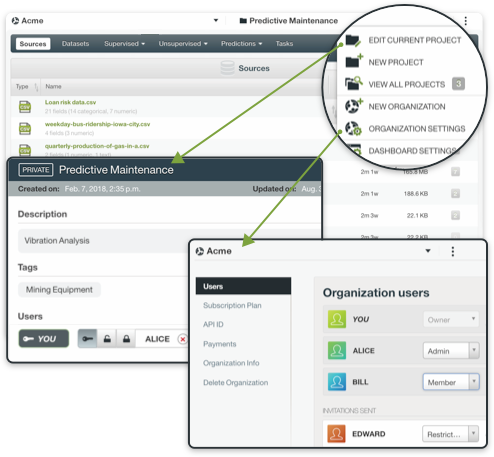
Collaboration
Share your Machine Learning resources using granular team and project management capabilities. BigML is a transparent, collaborative platform for all members of your organization, from analysts and developers, to engineers and executives.
Organizations: Effectively adopt Machine Learning across your entire corporate structure. With BigML organizations, the Dashboard is a shared workspace where users can access the same projects and resources with specific roles and permissions.
Projects: All resources in an organization exist within projects, which can be public or private. Assign user permissions according to the needs of each project, allowing other members to manage, create, or simply view resources.
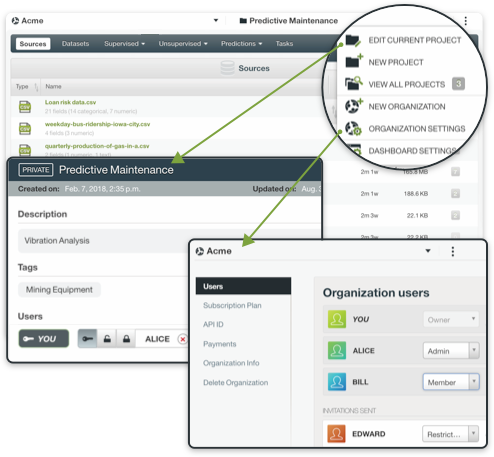
Programmable & Repeatable
As an "API-first" company, BigML brings every new feature first to the REST API. Bindings & libraries are available for all popular languages, including Python, Node.js, Ruby, Java, Swift, and more.
Reproducible: BigML's granular record keeping and transparency are crucial to meet regulatory and audit compliance requirements yet are often completely overlooked in other Machine Learning tools.
Traceable: All resources on BigML are immutable and stored with a unique ID and the creation parameters, which enables you to track any Machine Learning workflow at anytime.
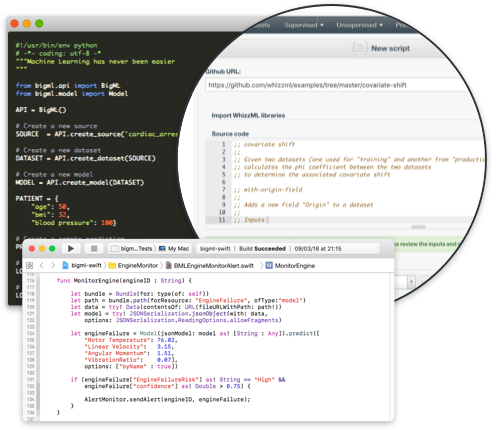
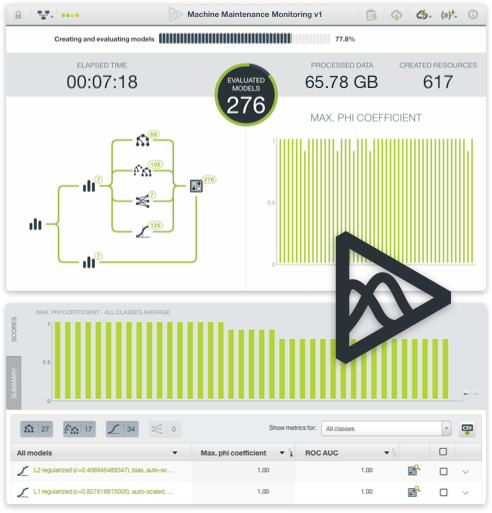
Automation
Rapidly bring your predictive modeling tasks to production through effective automation. BigML turns the difficult, time-consuming work of hand-tuning models or executing complex workflows into one-click menu options or single API calls.
OptiML: Automatic optimization for model selection and parameterization of classification and regression algorithms saves you a lot of time by creating and evaluating hundreds of models to find the best performing ones.
WhizzML: A domain-specific language for automating complex workflows, implementing high-level Machine Learning algorithms, and easily sharing them with others.
Scriptify: Convert your workflows into reusable scripts in a single click.
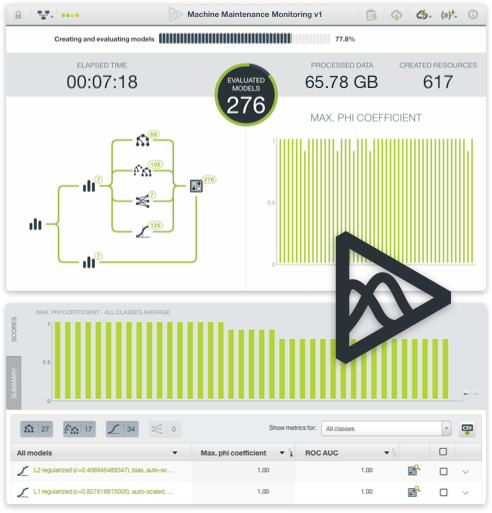
Flexible Deployments
Have tons of data? Need to provide access to everyone in your organization? Don't fret, all your bases are covered on BigML with options for multi-tenant and single-tenant versions on the cloud or on-premises. BigML can be ported to any cloud provider or to a Virtual Private Cloud, with fully-managed and self-managed versions.
BigML Lite: Fast track to value for companies ready to implement their first production use cases.
BigML Enterprise: Full-scale access for companies ready to adopt Machine Learning across departments.
Auto-scalable: All deployment methods utilize BigML’s smart infrastructure that automatically adjusts resources to seamlessly meet computational needs in the most cost effective manner.
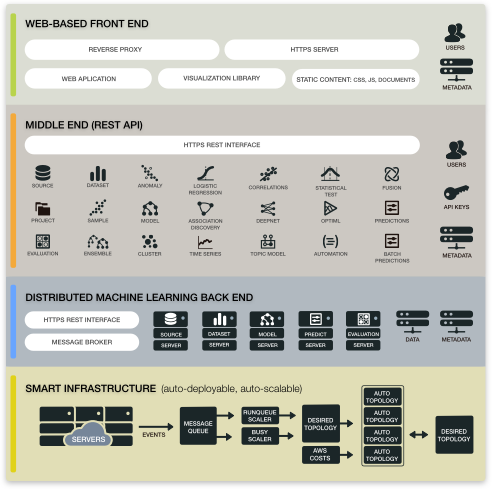
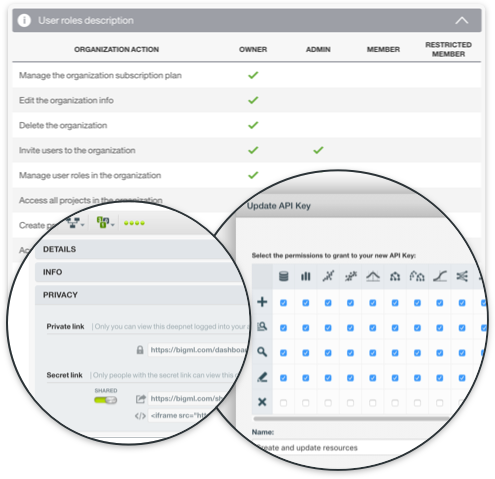
Security & Privacy
On BigML, every user has a private Dashboard and all resources created on it or via BigML's API are secure and private. All connections to BigML use HTTPS, ensuring the security of user data and communications. The BigML Team does not have access to any data in the system unless the user provides explicit consent.
Access privileges: Users can control who can view, edit and share at the resource level. Using organizations, you can assign different roles and permissions to others users at the project level.
Private Deployments: For companies with stringent data regulatory requirements, BigML offers two private deployment options that can run on your preferred cloud provider, ISP, or own infrastructure on commodity servers to meet the needs of your enterprise systems.
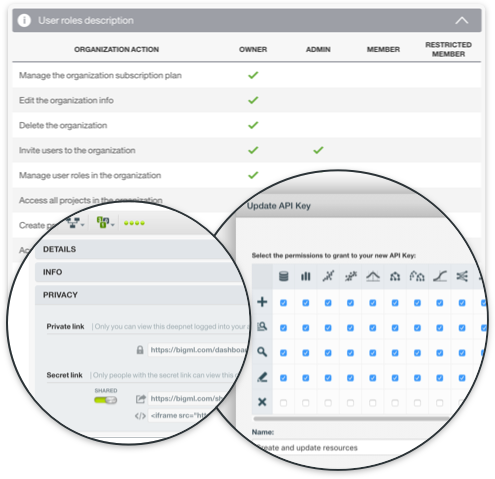
Integrate BigML's Machine Learning platform with your enterprise systems

Iterate and visualize Machine Learning models on BigML's intuitive, point-and-click web interface.

Build and integrate Machine Learning models using BigML's REST API and bindings in your preferred language.

Automate and share workflows with BigML's domain-specific language for Machine Learning.