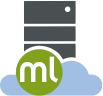
Real-time predictions in your own cloud
Do you need to make millions of predictions for your new data in real-time? The BigML PredictServer is a dedicated machine image which you can deploy in your own cloud to make lighting-fast predictions using your BigML models. PredictServer is available for customers with a Bronze or higher subscription plan or Private Deployment license as a Docker image.
Blazingly fast predictions
The BigML PredictServer keeps your models in memory and is optimized to make predictions quickly. In addition, the PredictServer can be deployed in your local network or in a Cloud region closer to your application to reduce latency.
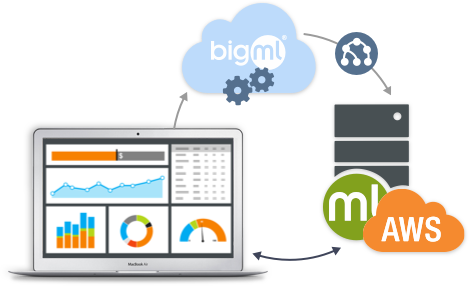
Highly scalable
Seamlessly integrate the BigML PredictServer with your existing applications and data center. The PredictServer performance scales with the CPU available. You can also instantiate multiple PredictServers to increase speed for larger amounts of data.
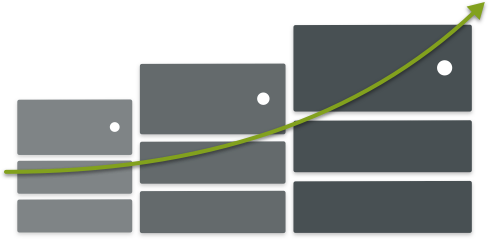
Easy to install and use
Deploy the BigML PredictServer in your own cloud. Cache models, ensembles, clusters or anomalies from BigML and make predictions via API calls. The PredictServer API is compatible with BigML's API and can be easily swapped in to support code integrated with BigML.
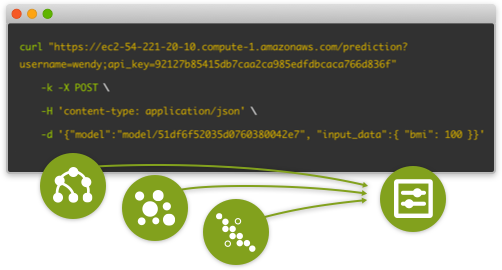
Quick start in 3 steps
1. Deploy the BigML PredictServer
Deploy the BigML PredictServer drectly from the image name you'll be provided with on the host where it will run. In the host console, execute the following commands.
docker pull provided-image-name
docker run --name carnac -v cache:/usr/local/carnac -p 8080:3000 -p 8081:3001 -p 8082:3002 -dit provided-image-name
Deploying BigML PredictServer
This will map internal ports 3000 thru 3002 to 8080 thru 8082 and mount the cache directory /usr/local/carnac at the host filesystem path ./cache
2. Change the auth_token
Change the auth_token
for the BigML PredictServer and add a list of BigML usernames to allow them access to the PredictServer. In the example below, we are changing the auth_token
to mytoken
and allowing the users wendy
and shara
:
curl "https://your-predict-server/config?auth_token=mytoken" \
-k -X POST -H 'content-type: application/json' \
-d '{"allow_users": [ "wendy", "shara" ]}'
Authorizing BigML users to access the PredictServer
3. Cache your models
Cache your models in PredictServer using the same username
and api_key
from bigml.io and then use them to make predictions:
curl -k -X GET "https://your-predict-server/model/51df6f52035d0760380042e7?username=wendy&api_key=92127b85415db7caa2ca985edfdbcaca766d836f"
Caching a model on the PredictServer:
curl "https://your-predict-server/prediction?username=wendy&api_key=92127b85415db7caa2ca985edfdbcaca766d836f"
-k -X POST \
-H 'content-type: application/json' \
-d '{"model":"model/51df6f52035d0760380042e7", "input_data":{ "education-num": 15 }}'
Predicting with your model